- Resource
- BR1DGE
- Early Detection
- Article
ATTD 2025 Symposium Summary
At the BR1DGE symposium at ATTD 2025, leading experts discussed how innovation can evolve the early detection and management of T1D (type 1 diabetes).
This article is intended to be a summary only. Additional topics were covered in the live symposium in line with the applicable regulations but are not included on the BR1DGE platform.
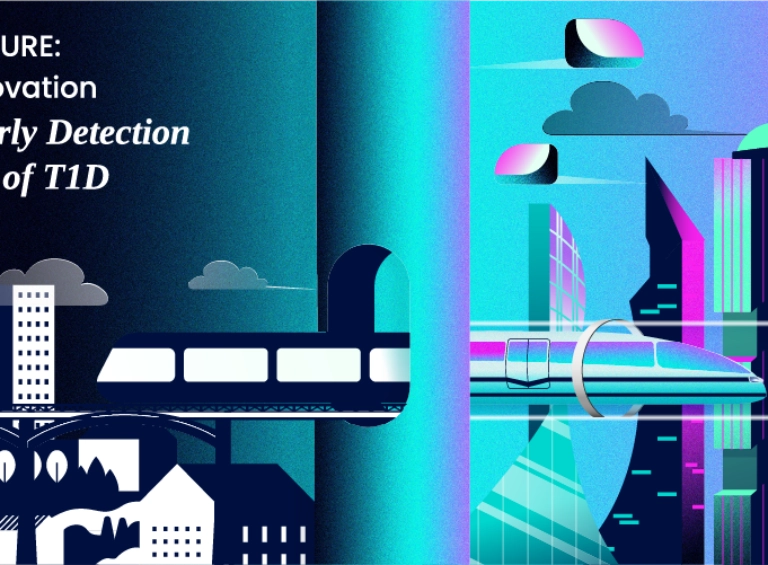
Meeting Objectives
-
Explore the current landscape and benefits associated with early detection of T1D as well as key challenges and unmet needs
- Discuss how innovations in islet autoantibody screening techniques, AI (artificial intelligence), and may enhance the benefits associated with early detection in the future
- Review latest strategies and how these may impact T1D management in the future
- Discuss the current challenges in the early detection and misdiagnosis of T1D in adults
Speakers
What Does the Future of Islet Autoantibody Screening Technology Look Like?
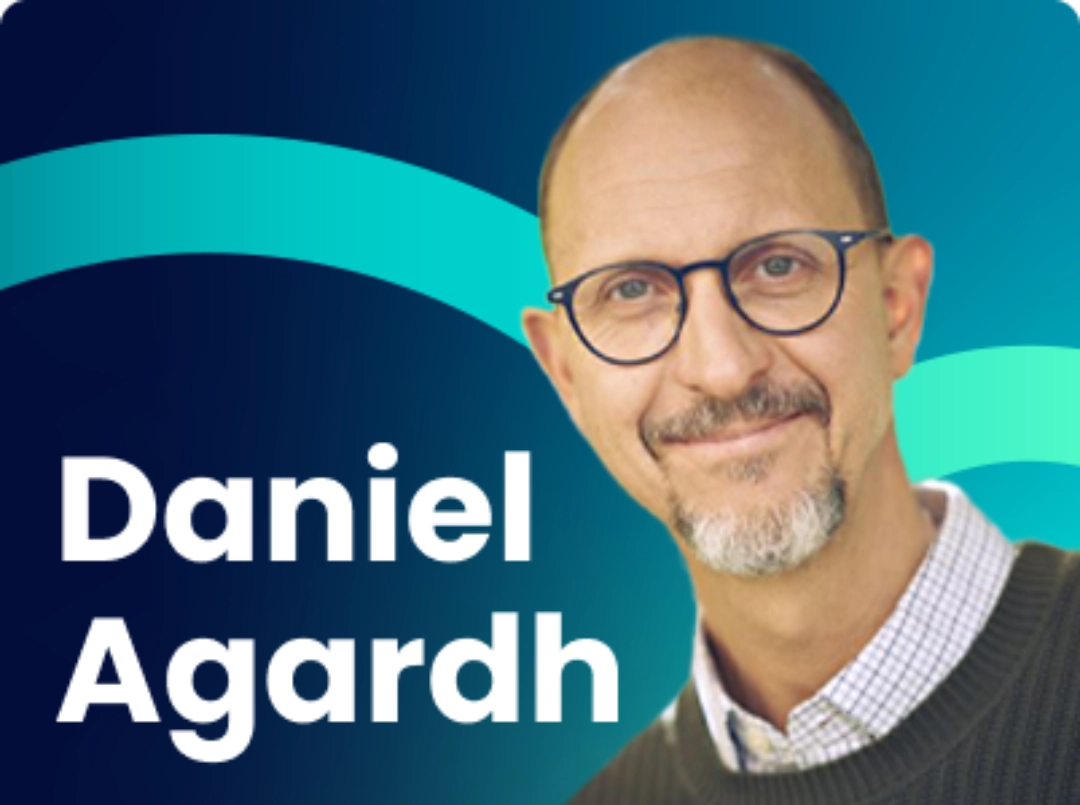
“Screening identifies people at risk for type one diabetes before clinical symptoms appear […] detection of islet antibodies IAA, GADA, IA-2A, ZnT8A is the key for the diagnosis and for early diagnosis.”
In the opening presentation, Prof. Daniel Agardh discussed advancements in screening for T1D, emphasizing the importance of early detection through islet autoantibodies.
- Screening helps identify individuals at risk before symptoms appear, especially children (ages 2–7 and 10–14) and adults with risk factors like a family history of T1D.1-4
- The four key autoantibodies— Insulin autoantibody (IAA), glutamic acid decarboxylase autoantibody (GADA), insulinoma-associated antigen 2 antibody (IA-2A), and Zinc-transporter 8 autoantibody (ZnT8A) —are essential markers for early diagnosis. Various assays are used globally, each with strengths and limitations.4
- Radio Binding Assay (RBA) remains the gold standard for islet antibody (IAb) screening. Radioactive-tagged antigen is used to bind to IAbs, the mixture is then filtered to separate bound from unbound antigens. The radioactivity of the bound molecules is measured to determine IAb levels.5,6
New screening methodologies offer viable alternatives to RBA and provide options for screening programs. These include:
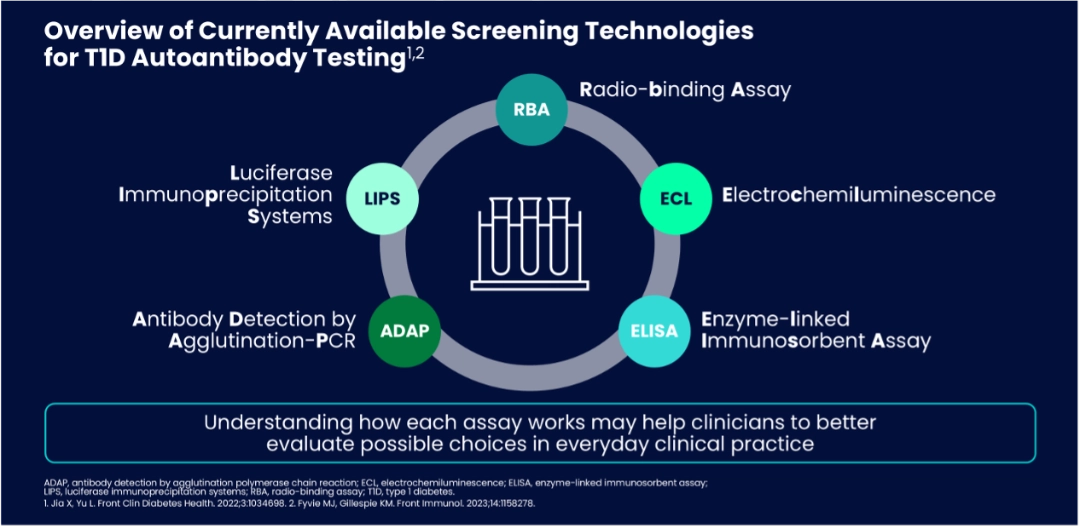
- Electrochemiluminescence (ECL) method is based on streptavidin -biotin and detection of bound antibodies by light emission from the sulfo-tag.7 ECL offers high sensitivity8 without radioactive material.9
- Enzyme-linked Immunosorbent Assay (ELISA) detects IAbs in serum sample using antigen-coated ELISA plate, where bound IAbs are quantified using a substrate-peroxidase colour reaction.10, 11 ELISA is cost-effective and widely used but less reliable for insulin autoantibodies.8
- Luciferase immunoprecipitation system (LIPS) assay uses Renilla luciferase (Ruc)-tagged antigens and protein beads that capture antibody-antigen complexes. Ruc-tagged complexes react with luciferin to produce light, which is measured to determine antibody levels.12,13 LIPS is sensitive for low-level antibodies.9,12,14
- Antibody Detection by Agglutination-PCR (ADAP) method uses DNA-labelled antigens to bind specific serum antibodies. Agglutinated DNA are amplified by PCR, and IAb levels are determined by measuring the amplicons. 15-17.
- ADAP is cost effective16, enables simultaneous detection of multiple autoantibodies9, and has potential for automation16 with low sample volume9 and with no radioactive material. ADAP has shown comparable or better performance than RBA in large studies, supporting its use in first-stage population screening.16
Real-World Implementation of AI to T1D Screening
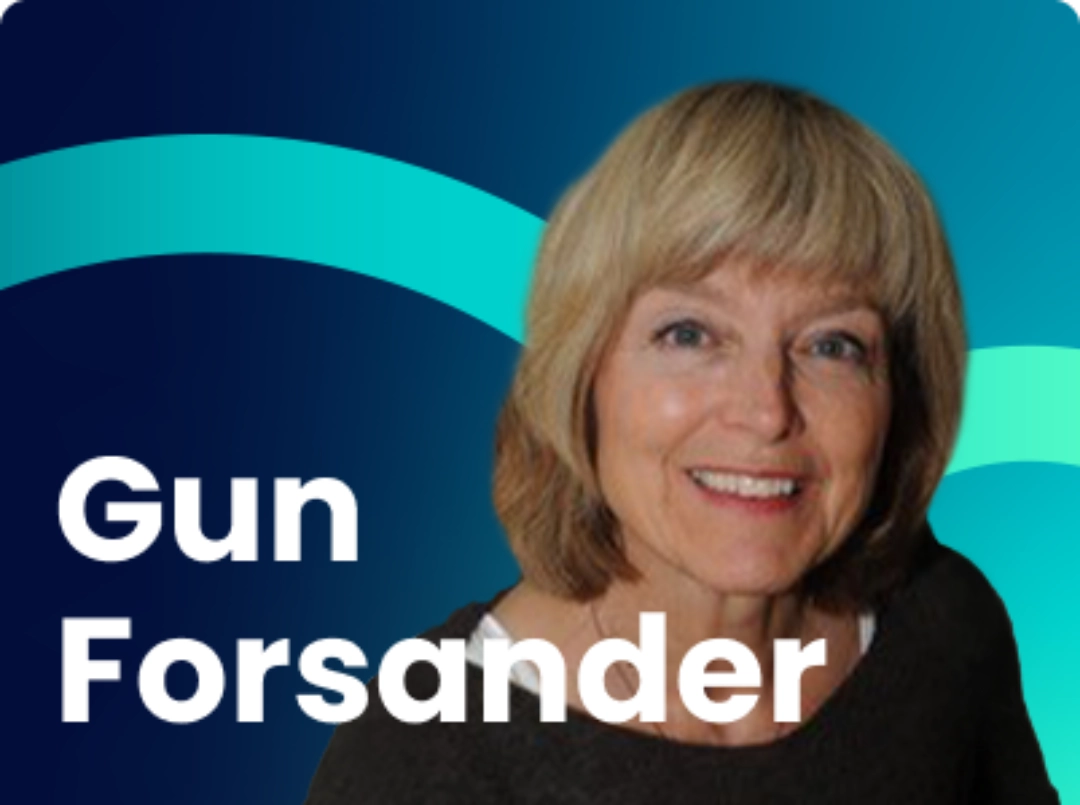
“AI algorithm in T1D can integrate diverse data sources from environment, genetics, biomarkers and variables and produce individualized risk scores, which is very interesting when discussing screening strategies.”
Dr. Gun Forsander discussed the role of AI in T1D screening, emphasizing its potential in risk stratification, biomarker identification, and selection of treatment based on predicted response.
AI generated risk profiles could be used to inform who to screen from the general population.
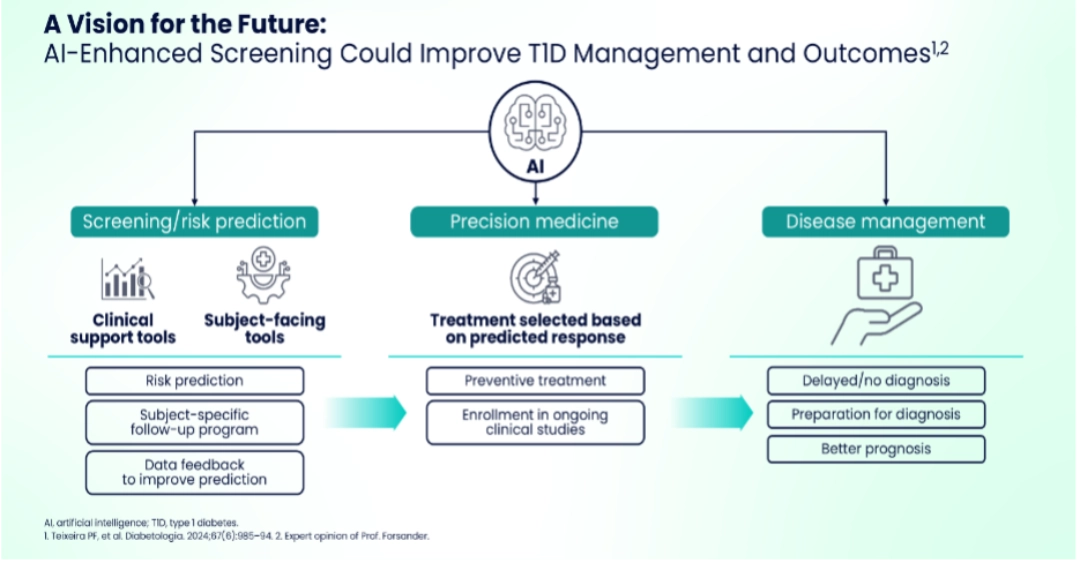
- AI has already shown promise in diabetes management through closed-loop systems and prediction and detection of complications.1-3
- AI for Sustainable Prevention of Autoimmunity in the Society (ASSET) Consortium in Sweden, uses the TEDDY dataset—tracking over 8,600 children at high-risk for up to 15 years—to develop AI models that predict individual progression to stage 3 T1D.4
- Results showed that data from three random visits provided up to 95% prediction accuracy, with islet autoantibodies and human leukocyte antigen (HLA) genotypes being key indicators. The AI model helped stratify individuals into high, intermediate, and low risk, enabling personalized screening and follow-up plans.5
- While the model developed has limitations such as focus on high-risk pediatric populations and data bases5, Dr. Forsander emphasized that AI-enhanced screening could improve T1D management and outcomes.4,6
What’s On The Horizon for T1D Management?
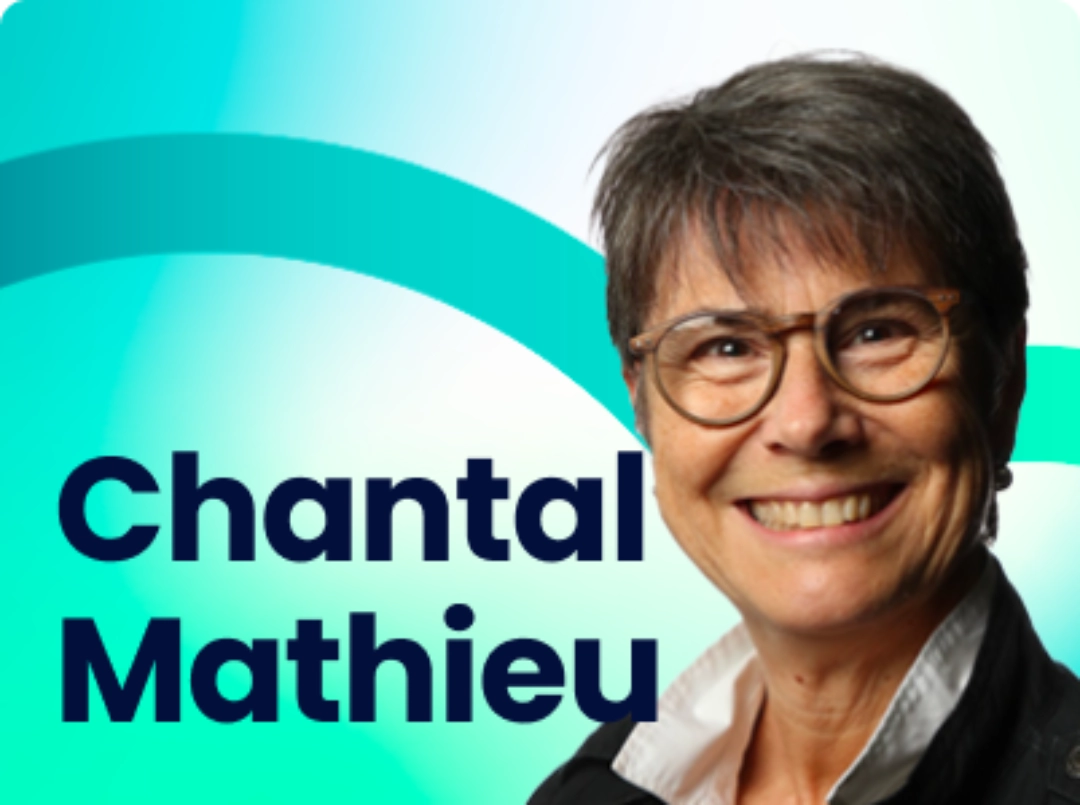
“We have to acknowledge that we still have long periods of the day, long periods of the month or the year where people with T1D are not under perfect glycemia control, exposing them to chronic complications - microvascular, macrovascular but, also to acute complications…”
Prof. Chantal Mathieu reviewed emerging interventions aimed at preserving beta-cell function in presymptomatic (stage 2) and newly diagnosed (stage 3) T1D.
The insight that T1D is an autoimmune disease and understanding its progression provides opportunities for therapeutic prevention strategies – at early-stage T1D having two or more autoantibodies, where risk of progression in children to stage 3 T1D is nearly a 100%; in stage 2 T1D, with two or more autoantibodies and dysglycemia, where the 5-year progression risk remains high (~75%); and stage 3 T1D with residual beta-cell function.
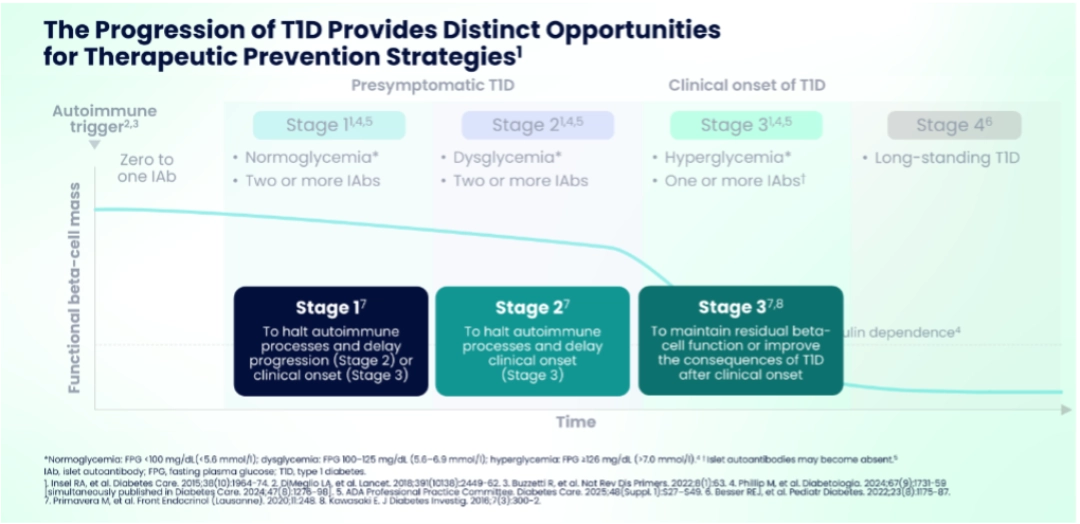
- Traditional approaches like insulin delivery systems (pens, pumps, and algorithms) aim to replicate beta-cell function but leave gaps in glycemic control, leading to chronic and acute complications.1-3
- Strategies aimed at slowing disease progression have shown the potential to extend endogenous insulin production, which can improve glycemic control and reduce complications.4-6
- Clinical approaches have demonstrated the ability to delay the transition from early autoimmune activity to full insulin dependence, offering individuals more time with residual beta-cell function.7-13
- Even for those with established T1D and minimal remaining C-peptide, advances in beta-cell replacement hold promise.14
- Emerging research explores ways to replenish insulin-producing cells while protecting them from immune destruction, either through encapsulation or immune modulation.14,15
- These innovations could transform long-term management, reducing the burden of intensive insulin therapy and improving overall metabolic outcomes.
Leave Nobody Behind: Early Detection and Diagnosis of T1D in Adults
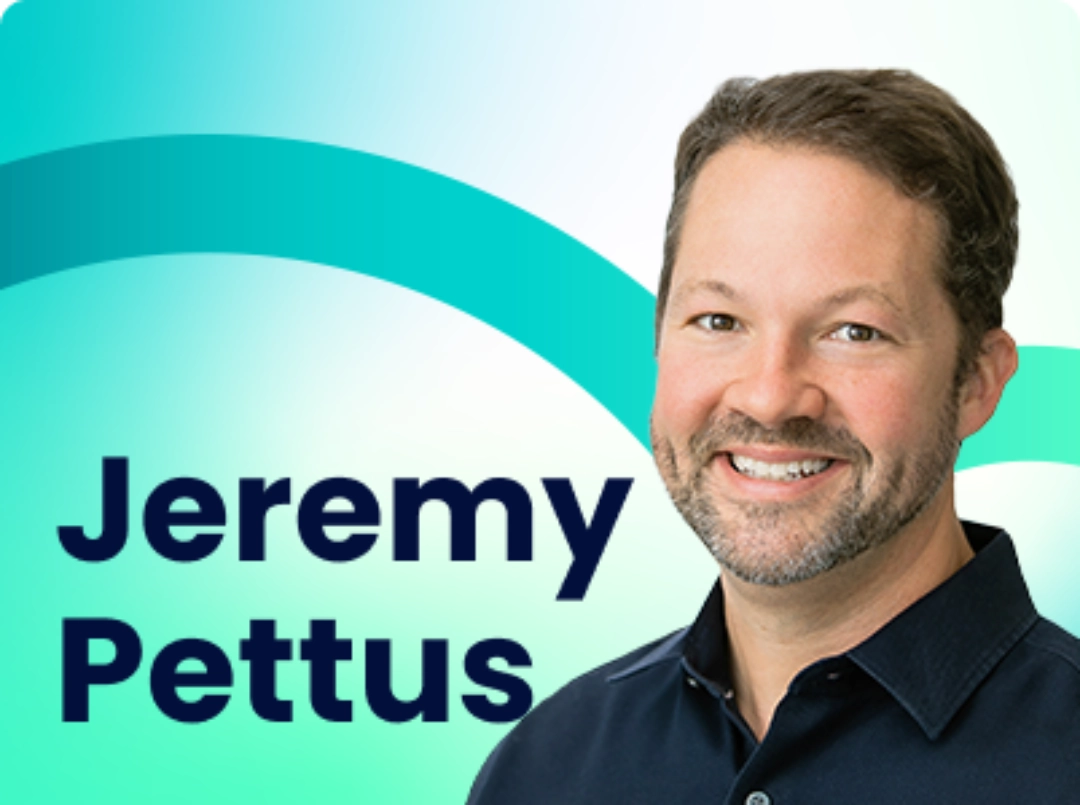
“We really need to be more aware that just because somebody is an adult doesn't mean it's automatically type 2 […] most people with type 1 diabetes are diagnosed as an adult.”
In the final session Dr. Jeremy Pettus discussed the current challenges in the early detection and diagnosis of T1D in adults.
In 2021, an estimated 62% of new type 1 diabetes diagnoses occurred in individuals aged ≥20 years.1 Up to 40% of adults with T1D are misclassified, with most initially diagnosed as type 2 diabetes (T2D).2,3
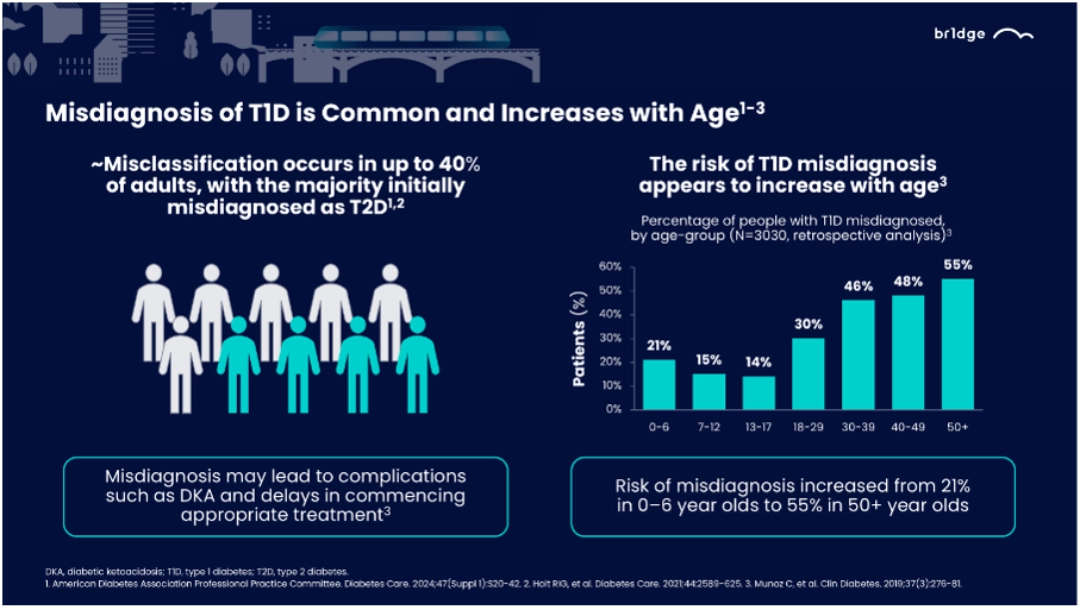
- Misdiagnosis of T1D is common and increases with age2,3,4
- Autoantibody testing and C-peptide measurements are critical tools to distinguish type 1 from type 2 diabetes, especially in cases where clinical characteristics are ambiguous.2,5,6
- Incorrect diagnoses delay appropriate treatment, leading to prolonged hyperglycemia and associated complications.
- Increasing clinician awareness and establishing systematic diagnostic protocols can reduce misdiagnosis. Emphasizing that T1D can occur at any age and recognizing its diverse presentations are crucial steps toward better patient outcomes.
MAT-GLB-2501901−1.0−04/2025
What Does the Future Of Islet Autoantibody Screening Technology Look Like?
- Besser REJ, et al. Pediatr Diabetes. 2022;23(8):1175-87.
- Sims EK, et al. Diabetes. 2022;71:610-3.
- Haller MJ, et al. Horm Res Paediatr. 2024:1-17. doi: 10.1159/000543035.
- Winter WE, et al. J Appl Lab Med. 2022;7:197-205
- Lind A, et al. eBioMedicine. 2024;104:105144.
- GiffordBioscience. https://www.giffordbioscience.com/radioligand-binding-assay/. Accessed March 2025.
- Gu Y, et al. J Vis Exp. 2018:133;57227
- Jia X, Yu L. Front Clin Diabetes Health. 2022;3:1034698.
- Fyvie MJ, Gillespie KM. Front Immunol. 2023;14:1158278.
- Delic-Sarac M, et al. Acta Inform Med. 2016;24(1):61-5.
- Kawasaki E, et al. J Diabetes Investig. 2023;14(9):1081–91
- Burbelo PD, et al. Tranl Res. 2015;165(2):325–35.
- Burbelo PD, et al. J Vis Exp. 2009;(32):1549.
- Jia X, Yu L. J Endocr Soc. 2024;8(1):bvad160
- Lind A, et al. J Immunol Methods. 2022;506:113265.
- Cortez FdJ, et al. SLAS Technol. 2022;27(1):26-31.
- Cortez FdJ, et al. PLoS One. 2020;15(11):e0242049
Real-World Implementation of AI to T1D Screening
- IEEE Spectrum. Available at https://spectrum.ieee.org/artificial-pancreas-could-conquer-diabetes. Accessed March 2025. 2. Guan Z, et al. Cell Rep Med. 2023;4(10):101213.
- AEYE Comprehensive Screening. Available at https://www.aeyehealth.com/aeye-comprehensive-screening. Accessed March 2025.
- Guan Z, et al. Cell Rep Med. 2023;4(10):101213
- Teixeira PF, et al. Diabetologia. 2024;67(6):985–94.
- Unpublished data from Prof. Forsander.
- Expert opinion of Prof. Forsander.
What’s On The Horizon for T1D Management?
- ADA Professional Practice Committee. Diabetes Care 2025;48(Suppl. 1):S181–S206.
- Singh A, et al. Eur J Cell Biol. 2023;102(2):151329.
- Hogrebe NJ, et al. Cell Stem Cell. 2023;30(5):530-48.
- Herold KC, et al. N Engl J Med. 2019;381(7):603-13.
- Teplizumab Prescribing Information. December 2023.
- Sims EK, et al. Sci Transl Med. 2021;13(583):eabc8980
- Quattrin T, et al. N Eng J Med. 2020;383(21):2007-17.
- Waibel M, et al. N Engl J Med. 2023;389:2140-50.
- Haller MJ, et al. Diabetes Care. 2018;41:1917-25.
- Haller MJ, et al. Diabetes. 2019;68(6):1267–76.
- Herold KC, et al. Nat Rev Immunol. 2024;doi:10.1038/s41577-023-00985-4
- Ramos EL, et al. N Engl J Med. 2023;389(23):2151-61 and Suppl.
- Forlenza GP, et al. JAMA. 2023;329(12):990-9 and protocol.
- Ho BX, et al. Front. Immunol. 2024;15:1375177. 2
- Hogrebe NJ, et al. Cell Stem Cell. 2023;30(5):530-48.
Leave Nobody Behind: Early Detection and Diagnosis of T1D in Adults
- Gregory GA, et al. Lancet Diabetes Endocrinol. 2022;10(10):741-60.
- American Diabetes Association Professional Practice Committee. Diabetes Care. 2024;47(Suppl 1):S20-42.
- Holt RIG, et al. Diabetes Care. 2021;44:2589-625
- Munoz C, et al. Clin Diabetes. 2019;37(3):276-81.
- Leslie RD, et al. Diabetes Care. 2021;44(11):2449-56.
- McKeigue PM, et al. BMC Med. 2019;17(1):165.